This is the second essay of three in a series arguing against anthropomorphizing LLMs in education. The other two are my exploration of questions about the educational value of historical chatbots and my review of Ethan Mollick’s Co-Intelligence.
For many, it seems natural, even inevitable, that we anthropomorphize large language models (LLMs). After all, they talk! Science fiction has taught us to expect talking computers to be like people. It can be fun to talk to a disembodied, very helpful, not-really-a person as long as it’s Her and not Hal. So far, entertainment seems to be what LLMs are good for.
Using them for work or for learning is different. In those contexts, pretending LLMs are people creates problems, not the least of which is that it obscures how they work and how they might help us do our jobs or learn new skills.
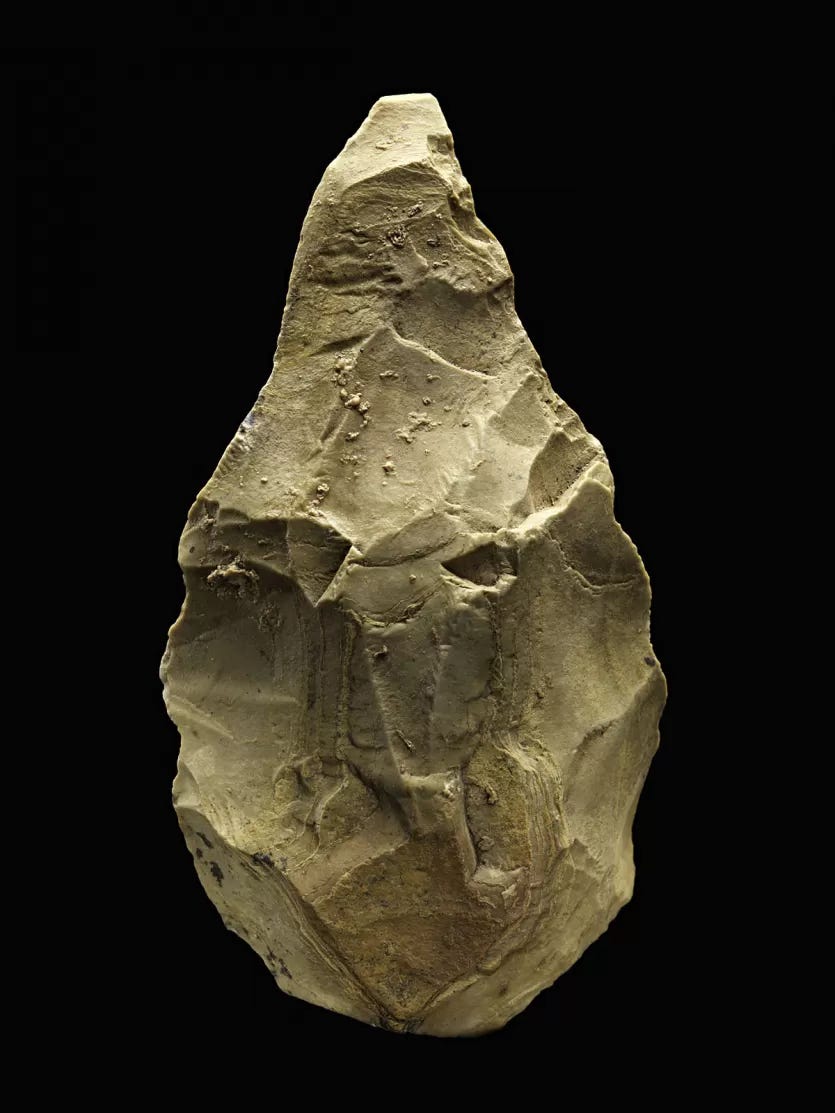
We talk to our tools. So what?
Anthropomorphizing tools is nothing new. A million years ago, humans saw faces in the moon as we used it to navigate the forest at night. This ancient handaxe looks friendly enough. I can imagine an ancient human unburdening himself like Tom Hanks talking to Wilson in Castaway. Our ancestors had one-sided conversations with their tools. Imagine a stone-age dad picking up that hand ax, looking it in the face, and telling it how the kids don’t appreciate his sense of humor. Maybe, I’m projecting? Maybe we’ve all been projecting when it comes to LLMs?
We never stopped talking to our tools. Lately, though, something changed. Now, our tools talk back. As the chatbot ELIZA demonstrated in the early days of digital computing, turning those ancient, one-sided conversations into something two-sided has weird effects on humans. ChatGPT was, as everyone likes to say, a game-changer. People really like playing the game of talking with machines that talk back. Sometimes, we get carried away.
Are we getting carried away when we act as though LLMs are people?
Games are an important context for thinking about this question. One way to understand LLMs is to see them as another successful attempt to build a game-playing computational intelligence. In 1997, we were amazed when Deep Blue beat Garry Kasparov at Chess, and again in 2016, when AlphaGo beat Lee Sedol in the even more complex game of Go. With ChatGPT, we now have a machine that can hold its own in the game of having conversations with humans.
Until recently, only humans could play these games. We may talk to our pets or at our cars, but we only talk with other humans.
Ludwig Wittgenstein called such interactions language games, and they are different than board games. For one, the goal of playing chess or Go is to win. Winning is not usually why we play language games, even though conversations can sometimes have winners and losers. Language games are mostly cooperative games with shared goals like exchanging information or establishing relationships.
Think about the way you greet your family or housemates in the morning. The game begins with “Good morning” or “How did you sleep?” and ends with “See you tonight.” or “Have a good day.” We play similar games in the office: “How was your weekend?” “Did you see the game?” is the opening move. These rule-based games have familiar patterns. Each player takes turns speaking. Feelings are expressed. Information is passed. The games end predictably with a final move like “Goodbye” or See you tomorrow.”
Until recently, only humans could play these games. We may talk to our pets or at our cars, but we only talk with other humans. And now we talk with computers. The people shoveling money into AI start-ups seem convinced that better natural language interfaces are something they can charge a lot of money for. We’ll see about that. I am more interested in figuring out the educational value of talking with talking computers.
Understanding how LLMs play language games should make us wary of treating them like people
I can see why there is such an enthusiastic response to a talking machine. LLMs seem to understand me. As anyone who has tried to get Siri or Alexa to do something knows, natural language processing has been a hard set of problems. The rules of language games are open-ended, and the patterns quite diffuse. The structure of the game has at least two players with goals guided by wide-ranging interests and intentions. When we engage in a two-way conversation, we assume, without thinking about it, that the words we speak are received by a mind capable of understanding and acting upon them. But that is not what happens when we prompt Siri or Alexa. And it is not what happens with an LLM.
Entering text into the context window of an LLM starts a computational process that establishes relationships among the words. The words that the LLM generates in response are, as Charlie Stross says, “a lump of text in the shape of an answer.” That lump is built out of statistical connections among words arrived at computationally, not intentionally crafted sentences meant to be understood. By responding intelligibly, an LLM creates the impression that it understands you. If you think it did, you are misunderstanding how the tool works.
In his book Co-Intelligence and his blog One Useful Thing, Ethan Mollick is clear-eyed about what LLMs actually are. He doesn’t see sparks of consciousness in LLMs or suggest that transformer-based generative models are a step toward AGI. His argument is practical. We need to give people a framework to start using them. What Mollick is arguing for is a suspension of disbelief, the habit of mind that lets us believe a character in a movie is a real person and that the plot is really happening. This “poetic faith,” as Coleridge called it, allows us to care about the fictional people and situations in stories we experience on stage or screen.
I’m not sure that poetic faith works the same way in the context of productivity or learning tools. What does it mean to suspend disbelief in a tool at work or at school?
Mollick points out that unlike traditional software, which at least aims at consistency, LLMs are weird and unreliable in ways that seem similar to humans. This is fundamental to their probabilistic computational structure. The idea is the skills and habits we have from playing language games with humans help us navigate the challenges of exchanging information with these weird computational models. Extending poetic faith to include the humanity of LLMs makes our use of them more efficient.
I’m proposing a pragmatic approach: treat AI as if it were human because, in many ways, it behaves like one. This mindset can significantly improve your understanding of how and when to use AI in a practical, if not technical, sense.
Most people don’t need to understand how LLMs work technically. They just need an effective way to get started. As Mollick says, “Working with AI is easiest if you think of it like an alien person rather than a human-built machine.” This makes anthropomorphizing an LLM a life hack, like using a clothespin instead of your fingers to hold a nail as you hammer or boiling salt water in a food-encrusted pot after you burn dinner. If you talk to an LLM like it is a person, you can leverage its ability to play language games to get it to understand instructions and produce better outputs.
I’m finishing up an essay on historical chatbots. To receive that essay and others about the social contexts of educational technology, please
Not everyone is excited to work with weird aliens who might take their job
The downside to embracing efficiency of use over understanding the technology you are using seems pretty obvious. To use a tool effectively, it helps to understand how it works. Pretending a transformer-based language model is a person obscures the technology’s shortcomings and its power. Computational intelligence is quite different from human intelligence, so much faster and weirder, especially if your framework is traditional software with its emphasis on consistency. Of course, human cognition is weird, too, but human weird is quite different from Golden Gate Claude weird.1
When I have expressed my skepticism about anthropomorphizing LLMs to AI enthusiasts, the response is often that I’m taking the downside of their approach too seriously. People are perfectly capable of pretending an LLM is human and understanding how its computational processes work. I think that’s probably true for some people. Look at all the early adopters who follow Mollick on LinkedIn or hang on to every word of the AI influencers who have been enthusiastically selling the AI future. For them, adding a layer of let’s pretend in order to learn a new tool sounds fun. If you are a fan of science fiction or fantasy, treating a human-built machine like an alien or magical being has great appeal. However, not everyone is going to be excited by pretending this weird and unreliable tool is an alien friend.
Little harm can come from treating a hand axe or an automobile as a conversational partner. When the tool talks back, the game really has changed, and we need to understand how.
This was brought home to me a few weeks ago at Explorance World, an ed-tech conference sponsored by a company that has been exploring machine learning for assessing learning for more than a decade. Kian Gohar gave an interesting talk before mine and made what was, for me, the unsurprising observation that saying words like please and thank you in prompts leads to better outputs from LLMs. If you had read Ethan Mollick or been playing around with LLMs, you would have learned this hack months ago.
The attendees were mostly technologists who work in higher education or human services but not in roles that relate to AI. As I engaged with the audience during my talk, I learned that most of them did not consider Kian’s suggestion an interesting hack. Rather, the idea that they needed to be polite to an LLM freaked them out a bit. It provided a glimpse of the verbal uncanny valley that was opening before them, a sense that the relationship between humans and tools was not what they imagined, that perhaps, in Thoreau’s words, humans “have become the tools of their tools.”
This reaction suggests the downside of the push to anthropomorphize AI. If you are not an enthusiast, then thinking of an LLM as a person may not sound appealing. The framework Mollick offers is “There's a somewhat weird alien who wants to work for free for you. You should probably get started.” To an early adopter who likes experimenting with new tech, that sounds great.
Most people are not so enthusiastic. They are tired of learning a new information system or productivity tool every few months. They may be anxious about their job or frustrated with the last big tech rollout at their company. Here is what they hear:
Meet your magic computer intern who can do a lot of your job for you. If you talk to it like it is a person, you will find it will be more creative, productive, and efficient. By the way, we think these interns will transform work so that only people who know how to talk to them effectively will be employable. You should probably get started. Be sure to be polite to it!
I can absolutely see the value of this framework for managers looking to motivate their workers through fear. I have no idea why knowledge workers or managers who want to build trust with their employees would see this framing as anything other than a threat. Anyone who has worked at a large organization understands that the pace of technological change is a challenge for workers. Maybe “copilots” and other natural language interfaces using LLMs will make productivity tools easier to use, helping to overcome resistance to yet another digital transformation initiative. If so, there are better ways to introduce it into the workplace.
In a recent essay on AI EduPathways, Mike Kentz suggests that in education, we should approach an LLM as if it were a brilliant stranger. “Stranger Danger” becomes the frame for managing the weirdness and unreliability of generative AI in the classroom. “But in order to do that,” he says, “you would have to view this technology as a human, with layers, a purpose that cannot be easily discerned, and motivations that are not always clear.” While I like the skepticism of this framework, I don’t like the way it obscures the reasons LLMs are unreliable. These tools do not have motivations, and their purpose is for teachers and students to determine. Better, I think, to treat them as complicated and interesting new tools that may or may not have educational value.
The problem with anthropomorphizing LLMs is that we blind ourselves to what this technology actually is: a form of computational intelligence fundamentally different from the human intelligence that built it. Little harm can come from treating a hand axe or the moon as a conversational partner. When the tool talks back, the game really has changed, and we need to figure out how.
Human intelligence built transformer-based language models, but that does not make LLMs co-intelligent
Another counter I hear is that we don’t know enough yet to do anything more than speculate. Computer scientists who build transformer-based technology don’t exactly know how LLMs are able to produce such compelling lumps of text. Some people think that it may be that human speech is simply the brain extruding lumps of text, too, and that human cognition can be understood as a more complex version of the computational processes in an LLM. If you believe that, then I can understand why you might think LLMs are intelligent and maybe why you’d get carried away by the idea that we are on a path to artificial general intelligence.
My simple argument for why human cognition is fundamentally distinct from whatever is happening in an LLM is to ask you to reflect on your own cognition.2 Think about the stream of your own consciousness. Ask yourself if the process of shifting from one thought to the next feels computational? Are you predicting the next word that comes out of your mouth based on vectors that give weights to specific relationships among words?3 If the answer is no, then you have reason to be skeptical of the idea that the processes in LLMs are similar to human cognition.
There are plenty of other skeptics. This essay by
published in the newsletter discusses computer scientist François Chollet’s ARC-AGI benchmark, arguing that it provides a better way to measure the intelligence of LLMs than using well-known cognitive tests designed for humans. The attention paid to Chollet’s ARC prize, announced back in June, is part of a general shift in the discourse. Astonishment at the outputs of LLMs is being replaced with sharp questions about their actual capabilities. As the press grows weary of chasing the AGI ball that OpenAI likes to throw for them, Chollet’s work pulls attention toward an analytical frame for understanding computational intelligence as something more than the ability to play language games.An intern who bullshits their way through an assignment is not helping. An assistant who swears they have booked a reservation in a restaurant that doesn’t exist is not making your life better. Humans can learn from their mistakes, but correcting an LLM simply produces a lump of text in the shape of an apology.
Chollet’s frame makes clear that LLMs are not performing anything like human thinking, even as ChatGPT and similar tools are changing our relationship with digital technology. LLMs make it easier to interact with information systems and databases. A much improved natural language interface is a true technological breakthrough. And it looks as though LLMs really can produce working computer code in a way that is valuable. But this is not co-intelligence.
Better to think of transformer-based language models as a computational cultural technology, sort of like a word calculator. Or, maybe a better description is that they are cultural artifact calculators. Not only do they produce lumps of text, they now produce lumps of images in the shape of pictures and lumps of sound in the shape of songs. They calculate an average cultural artifact based on an input, and each output is an approximation of the average. For generating computer code or an email to someone you don’t care about, that’s good enough. For other cultural uses, the jury is still out.
LLMs will never work like traditional software because they are based on the probabilistic computation of cultural data, much of it incoherent, biased, and wrong. Mollick says, “AI is not good software. It is pretty good people.” But the giant database that is the internet doesn’t represent humanity at its best. Much of the work going into improving LLMs aims to prevent the bad from surfacing.4
Let LLMs be weird their way and humans be weird our way
Like people, LLMs are unreliable. However, people are unreliable in specifically human ways. We trust humans to understand the social contexts of what is said, even if we don’t always trust the veracity of what they say back or that they are well-intentioned when they say it. In contrast, we should not assume that an LLM understands us because it can’t. Computational intelligence, as it exists today, has no way of making sense of the social context of words. Nor can it relate your inputs to sensory experiences of the world. This explains why it confabulates, or to use a less accurate term, it hallucinates.
The reason grammar nerds and AI skeptics prefer the word confabulation to hallucination is that hallucination means to perceive something that is not actually present, while confabulation means to make something up that is not true, usually in the context of telling a story or having a conversation. Humans are not always aware when they confabulate. Often, it happens without the conscious intention to deceive. A person adds a compelling detail to a mostly true story or says something that they want to be true with great conviction. When an LLM confabulates, it is simply shaping words into lumps of text that humans can understand, filling in gaps, or projecting confidence. This happens not because they intend to deceive but because LLMs are incapable of verifying their outputs.
Maybe there will be processes grafted onto transformer-based processes that correct an LLM’s outputs. Until that happens, it is hard to see how LLMs replace human knowledge workers at the scale many seem to expect. It is also hard to see the advantages of pretending an LLM is a person. An intern who bullshits their way through an assignment is not helpful. An assistant who swears they have booked a reservation in a restaurant that doesn’t exist is not making your life better. Humans can learn from their mistakes, but correcting an LLM simply produces a lump of text in the shape of an apology.
Instead of treating LLMs like people, let’s approach these tools as potentially useful new cultural technology that has the tricky ability to sound like it knows what it is saying even though it doesn’t. Understood as an interesting but limited form of computational intelligence, an LLM might turn out to do useful things. We will never imagine those uses if all we see is a projection of ourselves.
𝑨𝑰 𝑳𝒐𝒈 © 2024 by Rob Nelson is licensed under CC BY-SA 4.0.
Language itself is weird. As I was finishing this essay, the word weird took on a new valence for readers who follow US politics.
For a longer and more complicated version of why human cognition is quite different from what happens in transformer-based language models, check out my ongoing series of essays exploring how the ideas of Williams James help make sense of generative AI. Many have expressed similar arguments about why we shouldn’t anthropomorphize LLMs, going all the way back to Joseph Weizenbaum and the Eliza Effect. I hope as more people actually engage with LLMs outside of entertainment, the reasons this is a bad idea will become clearer. [This footnote was added five hours after the original post].
If you want to understand what all that means, read Timothy Lee’s excellent explanation of how LLMs work at Understanding AI.
This takes a great deal of applied human intelligence, mostly provided by underpaid laborers in the English-speaking Global South. Pre-training, the “T” in GPT, is essential for this, and the work must be done with care. Questions of how human intelligence shapes these tools and how their outputs are to be understood by humans must grapple with how the technology is developed and the weirdness and unpredictability of the data it is trained on.
Too many guardrails and limited data will exacerbate the problem that its outputs are unoriginal, bland averages. Too little attention to the human labor required to create them risks making the entire project of generative AI an exercise in the extraction of resources like diamond mining or oil drilling, except the resource being extracted is human intelligence.
Such questions, and others like the environmental costs, are critical to ask now, while there is still time to avoid basing the entire structure of AI development on a dynamic of rich consumers in the US reaping the benefits and inflicting the costs on the poor in other places.
"Little harm can come from treating a hand axe or the moon as a conversational partner. When the tool talks back, the game really has changed, and we need to figure out how."
Would that we could go back to the early days of our species and blog on developments in flint-knapping!
While LLMs may be the first technology to use words to speak to us, there's something about technology/skill/tools which influences their possessors. Don't you think the uranium & other non-verbal materials and processes in the labs of the Manhattan Project engineers were "talking" to them?