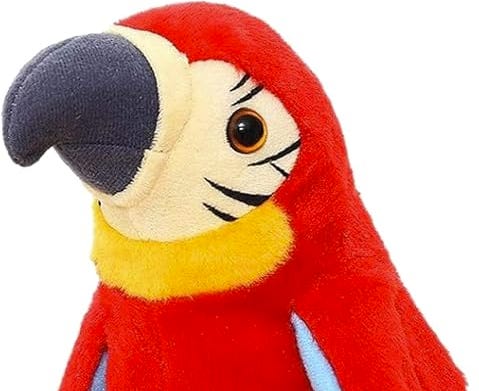
I enjoy Brad DeLong’s skeptical analysis of Large Language Models, but I thought it was a bit rude of him to use the phrase “utter bilge” to describe something Timothy B. Lee of Understanding AI wrote. Lee’s mistake was using the phrase “reason more effectively about abstract concepts” in reference to what LLMs may be capable of soon. Calling what Gemini or any LLM does “reasoning about abstract concepts” is anthropomorphizing LLMs to an extent that gives away too much to the con artists selling AI snake oil and the marketing professionals selling chatbots. Given the misdirection (or is it delusional thinking?) by OpenAI about what they are building and the religious beliefs about Shoggoths, paper-clip machines, and the nature of general intelligence that apparently pervade Silicon Valley, skepticism should be the rule when describing machine learning capabilities.
To his great credit, Lee tried to understand his error. He asked in the comments to DeLong’s post about how we can meaningfully distinguish between the information processes of LLMs and those of humans. As Lee put it succinctly: “I don't understand how you can be confident that the human brain isn't a more sophisticated ‘stochastic parrot’."
This is a good question! Since DeLong has not yet answered, I am going to give it a go.
…the best one-sentence answer to Lee’s question I have run across: “asking whether AI can be conscious in exactly the same way that a human is, is similar to asking whether a submarine can swim.”
There are three significant differences between the way humans and large language models use language.
Language is only meaningful in a social context. LLMs do not understand context, so they do not reason or think in ways that are remotely similar to humans.
Human brains are embodied; LLMs are not. This is an important difference because…
The complexity of the human brain is so much greater in terms of size and dimensionality that comparing them is a category error.
First, humans produce language; LLMs extrude text. This is the answer given in the paper that gave us the metaphor of stochastic parrots and that Angie Wang gives in Is My Toddler a Stochastic Parrot?. Language is social and no matter how sophisticated LLMs become, the language they generate will never be meaningful because, as Emily Bender and company put it:
Text generated by an LM is not grounded in communicative intent, any model of the world, or any model of the reader’s state of mind…The problem is, if one side of the communication does not have meaning, then the comprehension of the implicit meaning is an illusion arising from our singular human understanding of language (independent of the model).
A large model generates text and other outputs based on internal processes that have a few similarities to humans–they both use neural networks–but the outputs are meaningful only to humans. Meanings are socially constructed through understanding or attempts to understand what is said. Since all interactions between humans and GPTs are one-sided, only humans are performing the meaning-creating activities that we can call reasoning or thinking.1
Say what you will about actual parrots, but at least they are, like humans, complex, energy-efficient biological entities that can survive in the world. Foundational models are energy-hogging amalgamations of silicon chips that can simply be unplugged.
The second answer is that humans produce language using internal processes that are categorically distinct. To put it in machine terms, human hardware is fundamentally different from that of an LLM even if their outputs look the same. In a recent article in Nature, Anthony Cemero points to “the fact that humans are embodied, which is to say that we are living, biological creatures who are in constant interaction with the material, social, cultural and technological environment.” Because we are embodied, human production of language involves more than the brain. For example, systems for circulating oxygen and digesting food are deeply connected to the brain-centered processes we think of as producing and interpreting language. These processes were developed to successfully navigate a complex world by cooperating with a group of fellow humans. Say what you will about actual parrots, but at least they are, like humans, complex, energy-efficient biological entities that can survive in the wild. Foundational models are energy-hogging amalgamations of silicon chips that can simply be unplugged.
To borrow Angie Wang’s example, the word “cat” has sights, sounds, touches, tastes, and smells, along with memories and anticipations, that are associated with its use by a human.
Finally, the complexity of human information processing is so much greater than that of LLMs that to speak of them in terms of degrees of sophistication stretches the relation between the two past the point it breaks. Because they are embodied, humans accept a steady stream of experience through at least five senses from the moment they are born (let’s start there for simplicity's sake). Compare that to the meager input capabilities of even the latest multi-modal foundational models. LLMs do access more words through their training data—Cemero estimates that GPT 3.5 is trained on a dataset “with more than 5,000 times the number of words that a typical child hears”–but human language involves associations of words, not just with other words, but with a world of experience unavailable to GPTs.
To borrow Angie Wang’s example, the word “cat” has sights, sounds, touches, tastes, and smells, along with memories and anticipations, that are associated with its use by a human. That much complexity is orders of magnitude greater and qualitatively different than what an LLM can manage with no bodily awareness and no sense of time. Here is a paper, titled The Morphospace of Consciousness: Three Kinds of Complexity for Minds and Machines, that provides the best one-sentence answer to Lee’s question I have run across: “asking whether AI can be conscious in exactly the same way that a human is, is similar to asking whether a submarine can swim.” It is a doozy, so you might want to listen to Ricard Solé, one of the authors, talk about it on Sean Carroll’s Mindscape podcast. The authors seem to suggest that some form of machine general intelligence is possible someday far in the future, but their mapping of consciousness suggests that currently, LLMs are more like a message in a bottle bobbing on the surface of a pond than something capable of navigating the ocean’s depths.
I find these three answers compelling, but I don’t want to shut down those who disagree or have questions about LLMs and intelligence, consciousness, or linguistic practices. We should be exploring those questions, especially when they are asked in good faith. But we should also be careful not to provide cover to the bullshit artists trying to sell us on the astounding and mysterious capabilities of LLMs.
You can complicate this answer by following philosophers like Jacques Derrida and Richard Rorty into a linguistic skepticism that says there is nothing outside language with which to make meaning. This view would say that humans, parrots, and LLMs are all making moves in a language game in which players are producing language grounded, not in the experience of the world, but only in language itself. So, there is no way to distinguish an LLM’s model of the world from a human’s or a parrot’s because when it comes to using language, maybe it is stochastic predictions all the way down. If you find this position intriguing, I’m working on an essay about William James's view of consciousness that explores what circumstances would lead us to ascribe consciousness to machines. Due to answers #2 and #3, I think we are a very long way from encountering such circumstances, but the question is interesting and may be relevant for providing alternatives to those inclined to treat LLMs as gods in the making.