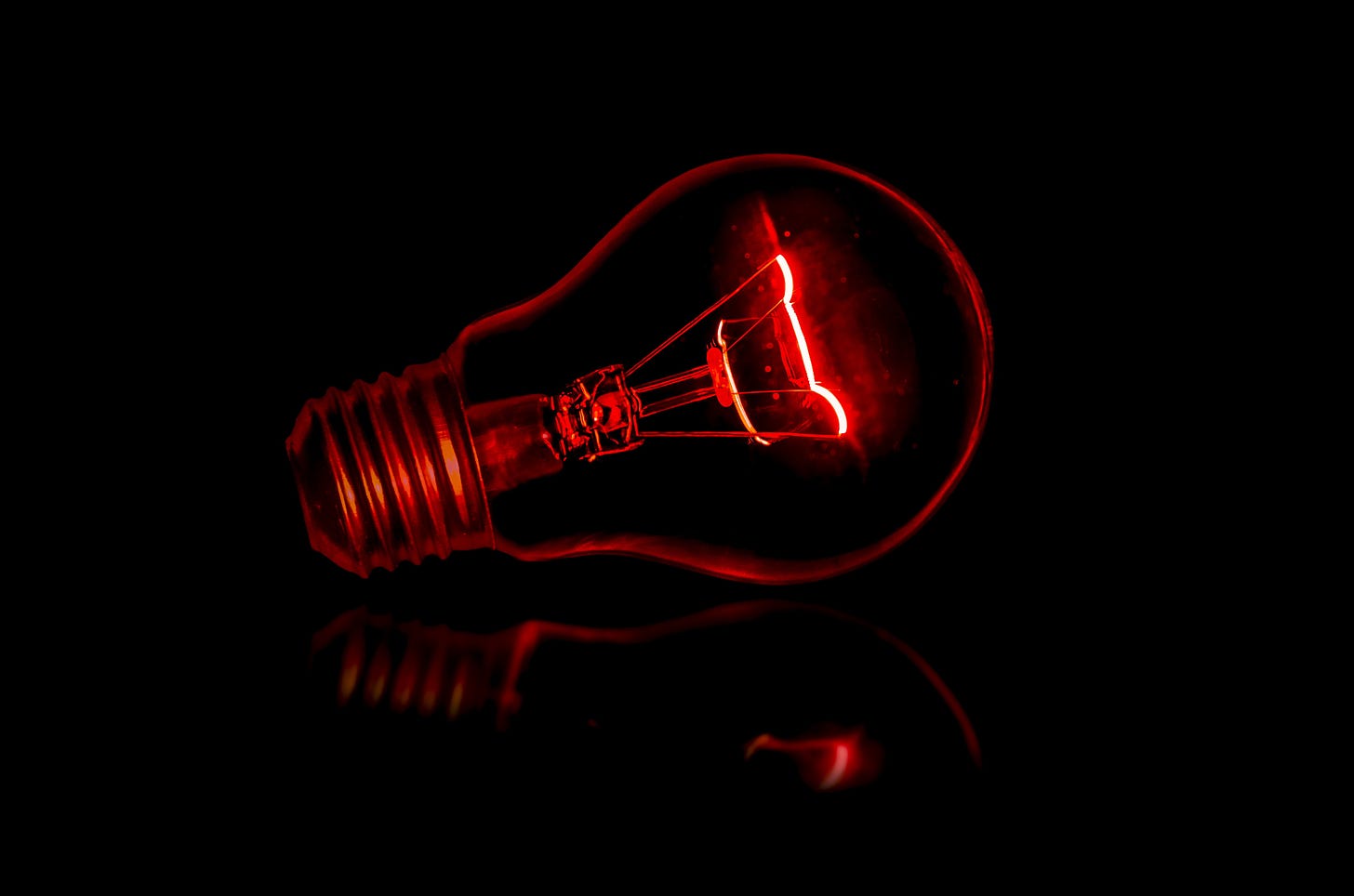
In my last post, I explored the question What have we learned about generative AI over the past year? Here I offer suggestions for educators about how to approach the coming year.
Put generative AI into social and historical context
Generative AI is one more new educational technology of the modern era, following the printing press and the pencil and continuing to the textbook and the electronic calculator. If we take the long view, we can even think about Large Language Models as building on the earliest educational technology: spoken and written language. A longer view may help reduce the urgency and confusion swirling around what can seem like completely unprecedented change.
Recognize that generative AI is something completely new
Every significant educational technology changes how humans learn, but adopting new tools takes time. The working out of practices made possible by generative AI will mean feeling our way along a passage dimly lit and with no clear idea of what lies before us. It will be unsettling and frustrating and will require abandoning teaching methods and administrative practices that have served us well.
Take care with the language we use
Much of the discourse about AI for the past year has been obsessed with comparing the outputs of machines to the outputs of humans. My favorite headline of the year was ChatGPT broke the Turing Test because it rightly characterized the problem as a lack of agreement on measuring the capabilities of generative AI machines, rather than panic about the model's ability to mimic human speech. Metaphors drawn from magic and science fiction can help understand these new machine capabilities, but they risk creating a fantasy world where chatbots are understanding friends or alien monsters, when in fact they are human-built tools that should serve human purposes.
Address the problems
Large foundation models built by OpenAI and Google have problems related to privacy, intellectual property, worker safety, ethical use of data, and environmental costs. These companies have become more secretive over the past four years, so our understanding of these problems has diminished even as the capabilities and use of the models have increased. As individual consumers and enterprise customers, we should hold technology companies accountable for addressing the problems and being transparent about their work.
Recognize the existential risk
No doubt, the breakthroughs in generative AI seen in large foundational models are a step toward artificial general intelligence (AGI). However, those closest to a new technology are often the most optimistic. The incentives for those close to cutting-edge research are to get investors excited, to be quoted in news articles, and to get your friends talking online and at parties. Many of those preaching end-of-days AI doom are millenarianian prophets whose incentives are similar to those of giant tech managers. The AGI optimists and P(doom) enthusiasts have one good point: the time to plan for the singularity is well before it arrives. So some attention is warranted, even if your P(doom) is well below 1%. However, the potential catastrophic harms in a vague and distant future should not distract us from solving the immediate problems of existing generative AI. We can and should address all harms, actual and potential.
Update your rules
We need rules against the bad things people do. When people do bad things with new tools usually the old rules still work to adjudicate wrongs. But maybe not? So colleges and universities need to review their rules and make sure they apply to the bad things people can now do with generative AI. Speaking of rules, here is a rule all colleges and universities need: Don’t let teachers try to use AI detectors to prevent students from using generative AI. AI detectors don’t work and they bias teachers who use them.
Engage students
In case you hadn’t noticed, college campuses are highly visible sites of intergenerational conflict. Attitudes toward using generative AI are one likely source of conflict as students use generative AI and some significant portion of teachers don’t. But there are others. The ethical and social problems with the largest foundational models are a potent mix of environmental, racial, and global concerns. Student activists may mobilize around one dimension of these concerns, or perhaps AI itself will become a focus of a new social justice movement. Whether that happens or not, we should ensure that students have a voice in the decisions we will be making.
See the global context
LLMs speak with an American accent and image generators process visual inputs through the equivalent of American eyes. That is because the datasets and reinforcement learning that determine their outputs are almost entirely in English, drawn from North American culture, and informed by North American values. Rest of World captures this truth in the strangely skewed visual outputs of Midjourney. The commercial, regulatory, and educational work with AI outside the United States, especially in China, the European Union, the United Arab Emirates, and Singapore is another important context often missing from the discourse.
Look past ChatGPT
The reinstatement of Sam Altman makes it seem less likely that ChatGPT will suffer the fate of MySpace and Napster. Yet the stumbles and restructuring of OpenAI are a reminder of how uncertain the AI future truly is. Even when measured in months. When Google releases Gemini, when Microsoft's co-pilots are actually being used by AI novices, and when other companies working on AI release something new, then the maps will be redrawn and we may find ourselves in a world where ChatGPT and the GPT platform are known primarily as a case study in the dangers of moving to commercialize a product too quickly.
Get ready for it
If you believe in human agency, and that we are the authors of our future, then the biggest challenge to building an AI future that supports human flourishing is a social psychology that inhibits action. Feeling overwhelmed by social and technological change, we feel a sense of helplessness and retreat. What can we do that would make a difference in the larger scheme? What’s the point of learning a new tool when it is all going to change again next year? Rational argument does little to change minds protected by mental armor against the rapid and unsettling changes of modern life.
The antidote to this dilemma is found in feeling. Feelings of love, of joy, of human connectedness, of abandonment to something new. Music has affective power whereas narrative and argument do not. To prepare for the coming year, I offer the most popular artist of the moment, singing as a human preparing for a new love, not as an innocent, but as a fully aware agent knowing it could all go wrong and taking the leap anyway.
Taylor Swift’s music video creates a vibe of human potential, augmented by technology, in a world of threats and uncertainty. It is an anthem for our future as we start another year of making choices about how to use generative AI.
𝑨𝑰 𝑳𝒐𝒈 © 2023 by Rob Nelson is licensed under CC BY-SA 4.0.